Trending Headlines
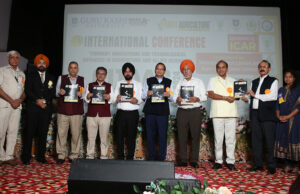
8th International Conference, Bathinda (CITAAS-2024)
Just Agriculture Edu. Group in collaboration with Guru Kashi University, Talwandi Sabo, Dept of Agriculture & Horticulture (Punjab Govt), ISASTR, Noida successfully organized 𝐈𝐧𝐝𝐢𝐚’𝐬 𝐋𝐚𝐫𝐠𝐞𝐬𝐭
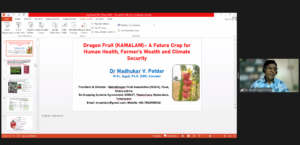
15 Day’s International Training cum Workshop Program- AGRICULTURE 1.0
15 Day’s International Training cum Workshop Program- AGRICULTURE 1.0 “Advances in Agripreneurship & skill development for reshaping future of Indian Agriculture” from 01 Aug-15 Aug,

15 Day’s International Training cum Workshop Program- AGRICULTURE 2.0
15 Day’s International Training cum Workshop Program- AGRICULTURE 2.0 “A Next level approach towards sustainability, smart farming & Agri-Innovation” from 16-30 Dec, 2021 which was
Get Trained By Us
Join our mailing list to receive the latest news and updates from our team.
We request you to go through the website properly as the website is self explanatory. If you need details of our editors, you can refer to our Editorial Board Page
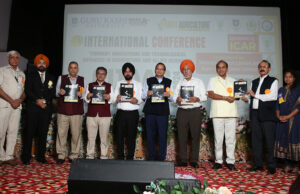
8th International Conference, Bathinda (CITAAS-2024)
Just Agriculture Edu. Group in collaboration with Guru Kashi University, Talwandi Sabo, Dept of Agriculture & Horticulture (Punjab Govt), ISASTR, Noida successfully organized 𝐈𝐧𝐝𝐢𝐚’𝐬 𝐋𝐚𝐫𝐠𝐞𝐬𝐭
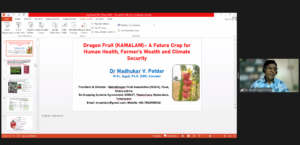
15 Day’s International Training cum Workshop Program- AGRICULTURE 1.0
15 Day’s International Training cum Workshop Program- AGRICULTURE 1.0 “Advances in Agripreneurship & skill development for reshaping future of Indian Agriculture” from 01 Aug-15 Aug,

15 Day’s International Training cum Workshop Program- AGRICULTURE 2.0
15 Day’s International Training cum Workshop Program- AGRICULTURE 2.0 “A Next level approach towards sustainability, smart farming & Agri-Innovation” from 16-30 Dec, 2021 which was
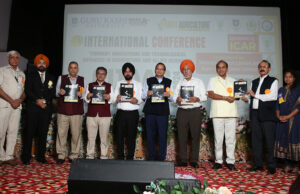
8th International Conference, Bathinda (CITAAS-2024)
Just Agriculture Edu. Group in collaboration with Guru Kashi University, Talwandi Sabo, Dept of Agriculture & Horticulture (Punjab Govt), ISASTR, Noida successfully organized 𝐈𝐧𝐝𝐢𝐚’𝐬 𝐋𝐚𝐫𝐠𝐞𝐬𝐭
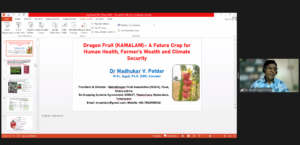
15 Day’s International Training cum Workshop Program- AGRICULTURE 1.0
15 Day’s International Training cum Workshop Program- AGRICULTURE 1.0 “Advances in Agripreneurship & skill development for reshaping future of Indian Agriculture” from 01 Aug-15 Aug,

15 Day’s International Training cum Workshop Program- AGRICULTURE 2.0
15 Day’s International Training cum Workshop Program- AGRICULTURE 2.0 “A Next level approach towards sustainability, smart farming & Agri-Innovation” from 16-30 Dec, 2021 which was
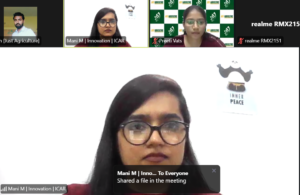
3 Day’s National Workshop “Sustainable utilization of Agricultural Resources” from 11-13 March, 2022
3 Day’s National Workshop “Sustainable utilization of Agricultural Resources” from 11-13 March, 2022 organised by School of Agriculture, Quantum University, Roorkee & Just Agriculture Education
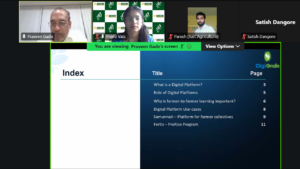
One Week International Faculty Development Program
One Week International Faculty Development Program on “Enhancing the ability of Quality Writing of Research Project & High Impact Publication” on 11-17 April, 2022 in
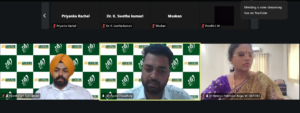
21 Day’s International Training cum Workshop Program- AGRICULTURE 3.0
21 Day’s International Training cum Workshop Program- AGRICULTURE 3.0 “A futuristic approach towards Smart Agriculture & Sustainable Urban Farming” from 30 April- 20 May, 2022
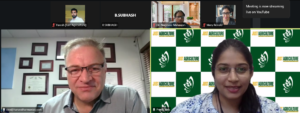
15 Day’s International Training cum Workshop Program- AGRICULTURE 4.0
15 Day’s International Training cum Workshop Program- AGRICULTURE 4.0 “The Future of Farming Technology & Agripreneurship Development” from 10-25 Nov, 2022 which was jointly organised
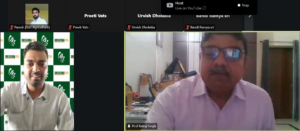
7 Day’s Online Training Program on Drone Manthan from 20-26 Nov, 2022
7 Day’s Online Training Program on Drone Manthan from 20-26 Nov, 2022 jointly organised by NAHEP-DFSRSDA-ICAR, VNMKV, Parbhani & Just Agriculture Education Group
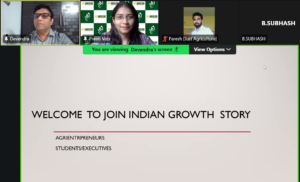
One Week International Faculty Development Program
One Week International Faculty Development Program on “Improving the Writing Skills of Early Career Researchers” on 15-21 Dec, 2022 in collaboration with SKUAST, Kashmir &
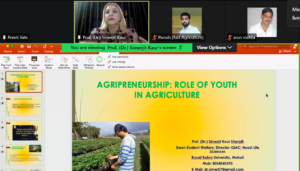
One Week Faculty Development Program
One Week Faculty Development Program on “Effective Research Methodology & Publication” on 13 Feb-19 Feb, 2023 in collaboration with SGT University, Gurugram & Just Agriculture